Three Steps We Took to Cultivate a Data Solution Portfolio
Successful data teams not only need strong data analysts, but they also need a strong product and project management foundation.
One in six machine learning models never make it to production. Often, it’s not because of faulty data or a lack of expertise, but basic project management. Many data teams are relying on disjointed, reactive waterfall methods. It's a fundamental gap that exists across many organizations where there’s a laser focus on execution, but little focus on the underlying planning and management.
Successful data teams not only need strong data analysts, but they also need a strong product and project management foundation. This foundation ensures that resources are aligned to business objectives, projects are properly prioritized, and stakeholders get the data needed to be successful. Ultimately, what data teams need is a framework that encompasses product management, project management and technical execution. So, that’s what we did and here’s how we did it.
Step 1: Lay the foundation
At Rackspace Technology, we took an innovative approach to our global data office. Our approach centralizes traditional concepts and incorporates them into the unique needs of our users. By adopting this method we’ve achieved a 7x increase in projects handled, from 14 projects per quarter to 91 per quarter, in addition to measurable success in velocity, on-time delivery and data model delivery timelines.
What we implemented is unique in three ways:
- Internal product management: The product management office vets projects, collects requirements and sets the priority. Our two-tiered model revolves around designing products and roadmaps for internal users so that they can provide better experiences and business value to our external customers.
- Project management: It's a fundamental shift in how we plan and execute projects. This can be a big change for organizations where functional leaders typically set the priorities and timelines. The project management function takes the priorities and roadmaps developed by the product managers and lays them into a timeline with milestones and check-ins so that functional teams can focus on delivery instead of management tasks.
- Scaled Agile: What further differentiates our approach is the use of Scaled Agile. It lets us take a problem and divide it into bite-sized chunks to execute against. Because data projects have so many components that get overlooked, Scaled Agile is ideal as it takes an end-to-end view of a project and supports the iterative nature of data projects.
With these best practices as our foundation, we infused our project pillars. Based on corporate objectives, these pillars define projects as those that deliver internal optimization, enable the business or transform the business. From there, we divided the portfolio into three parts, or products:
- Data-as-a-service: How we consume data. Includes business intelligence and advanced analytics.
- Data platform-as-a-service: How we centralize and harmonize data. Includes our infrastructure and data ingestion methods.
- Data management-as-a-service: How we establish trust. Includes data governance and master data management (MDM).
Within each product, we focus on projects that support our pillars to create a balanced portfolio that directly ladders up to our corporate objectives.
Alongside our portfolio are strict prioritization protocols led by business value. We study our backlog to find common threads. Instead of solving one problem for one business unit, we're trying to solve for the common threads across the business. This allows us to satisfy more users at once, streamline resources and make a bigger enterprise-wide impact.
Another important aspect to the framework is delivering a minimum viable product (MVP) for a functional solution. In a traditional development framework, the organization could wait months for a full release during which the project requirements have likely changed. In our framework, we meet with the stakeholders to determine the MVP and plan incremental releases to achieve the full bells and whistles. Starting with the MVP allows us to make iterative improvements, add value on a regular cadence, and stay close to changing requirements.
Step 2: Organize the team
Success hinges on finding the right leader. You don’t need a project management guru or data genius. Look for a person with strategic vision, business acumen and the ability to stand their ground. Accordingly, your team should consist of people that play well in both worlds — able to translate business needs to the technical side and vice versa.
Once we placed the right leader and assembled the team, we began by establishing clear roles and responsibilities. Then, we created a dedicated pod for each value stream that includes product managers, Scrum Masters and business systems analysts.
At that point, we scheduled in-depth working sessions. In those sessions, we attacked the backlog by tagging each request to a value stream and assigning business value. Next, we prioritized each project and developed a working roadmap. And we did all of this starting from zero to fully ramped up in just 60 days.
Each quarter, we take a few days to meticulously prioritize, plan the details and weigh dependencies for what’s next. Projects are locked in for the next quarter and then we start executing. As we shifted left, we started driving velocity, which accelerated project delivery, created more demand and further established trust.
Step 3: Reap the benefits
Once implemented, we quickly saw improvements in some of our key metrics. And we did this, not by adding people or throwing money at it, but with better planning and putting the right people in the right roles.
The numbers speak for themselves:
- 7x increase in projects from 14 projects per quarter to 91 projects per quarter
- 20% increase in velocity without adding headcount
- 80% year-over-year on-time delivery up from 15% on-time delivery
- 14 days to develop a data model, down from 91 days
Using our approach, we’ve reached maturity in our Customer Churn and NPS (customer satisfaction) product lifecycles.
Integrating product management into the data office has made us more reliable and more of a partner to the business. Our goal is to become even more proactive with our ear so close to the business that we’re able to get projects started before the business asks for it.
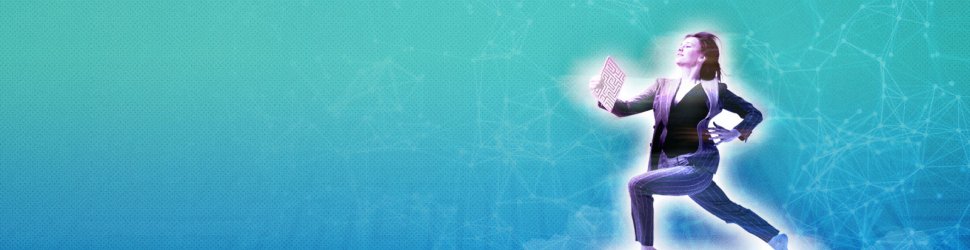
Tackling AI and Machine Learning’s Biggest Barrier – Data Modernization
About the Authors

Chief Information Officer
Juan Riojas
As Chief Information Officer at Rackspace Technology, Juan Riojas is responsible for enterprise-wide data strategy, management, and analytics to meet the need of the business to answer critical questions through time to insight. He has more than 20 years of industry experience successfully migrating data ecosystem across all public clouds, leading to significant business transformation outcomes. Prior to Rackspace, Juan worked for Informatica building their inaugural Data Office and has held various executive leadership roles at Gogo, Dell, Accenture, and Expeditors. A native of Texas, Juan attended Texas A&M International University, where he studied business administration and holds a post graduate degree from Said Business School, Oxford University
Read more about Juan Riojas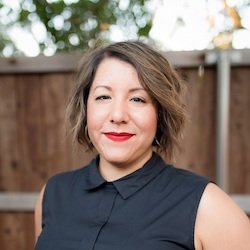
Director of Product Management
Lara Indrikovs
Lara Indrikovs serves as the director of product management for the Global Data organization at Rackspace. She is responsible for leading the development, management, prioritization and execution of the GDO product portfolio that encompasses end-to-end enterprise data initiatives. Lara is a certified SAFe leader and led the GDO’s agile transformation. Prior to her current role, Lara led the marketing intelligence and data science teams, which were dedicated to developing actionable reporting and insights for Rackspace’s marketing activities and creating predictive analytic tools to enable business transformation initiatives. In that role, she led the global migration and consolidation of web analytic platforms to Google Analytics 360, directed the Salesforce Cloud and Google Analytics 360 integration, and established the first Marketing datamart in GCP Big Query. Before Rackspace, Lara held various leadership positions at global advertising agencies, where she focused on multicultural media strategy and buying. She graduated from the University of Texas at Austin with a degree in Advertising and Media, later earning a Master of Science in Predictive Analytics from Northwestern University. Lara lives in Austin with her fiancé and 8-year-old Cocker Spaniel, Indiana Bones. In her free time, she likes to balance out her analytical left brain on a yoga mat, traveling, hosting dinner parties, dancing Rumba and other Afro-Latin dances and volunteering with local non-profits.
Read more about Lara IndrikovsRelated Topics