Enhance Your Human Talent with AIOps
AIOps shows that the technology has the potential to enhance — rather than undermine — the role of humans in the workplace.
A complex mix of optimism and uncertainty often accompanies significant technological disruption. And right now, no technology promises – or threatens, depending on your viewpoint – more disruption than artificial intelligence (AI).
For each expert who celebrates its transformative potential for both businesses and wider society, another will sound the alarm about the risks to jobs when machines start making decisions. Not to mention the moral implications when those decisions impact people’s lives and livelihoods.
These reactions are understandable, and there’s much to discuss as we move down this path. However, as AI applications begin to take root in enterprises (mainly through machine learning, a subset of AI), we’re already starting to see opportunities for it to enhance, rather than undermine, the role of humans in the workplace.
By reducing the noise in the tidal wave of data that businesses and technology now generate, AI systems have the potential to deliver higher quality information to teams, and to do it faster. With the deeper insights that can be extracted from that information, businesses can make better decisions and offer better recommendations to customers.
AIOps blazes a trail for machine learning
IT operations is one of those areas where we’re beginning to see the value that machine learning creates, thanks to the emergence of AIOps.
In today’s increasingly complex modern IT environments, especially where the cloud is concerned, domain-centric monitoring and management makes it difficult (or impossible) to gather the insights needed to be anything other than reactive to system issues.
What is AIOps?
By contrast, AIOps looks to automate the discovery process across IT operations functions and apply machine learning to detect patterns and make recommendations. This means that in addition to better visibility into availability, performance, service management and automation, teams can gain a sense of what lies ahead through event correlation and analytics capabilities.
AIOps empowers IT support teams to move beyond pushing tickets to becoming collaborative and creative problem solvers. Machines are unlikely to learn collaboration and problem solving anytime soon, but the analytics provided by AI can make an engaged IT team a problem-solving powerhouse.
How will AIOps affect employees?
Of course, AIOps will come with some level of disruption to workers, and unique challenges for people managers. AIOps means support jobs will soon look very different from how they look today. The upside is that by letting machines handle the data, and provide deeper insights into complex programmatic and mechanical relationships allows people the opportunity to focus on customer outcomes.
Knowledge sharing: How Rackspace uses AIOps
This viewpoint is based on our own experience of AIOps, which has allowed our support teams to do less triage work and provide more service by replacing single alert tickets with grouped “situation” tickets.
Traditionally, an issue with a single system may trigger alerts in multiple functional areas and fire a ticket out to each of the teams responsible for that domain (such as storage, network, virtualization, OS and applications).
Now however, we’ve adopted the Moogsoft AIOps solution, which uses supervised machine learning systems with “knowledge” of our environments’ topologies to spot patterns and correlations in support issues. For example, we might simultaneously get web alerts, server availability alerts and OS alerts that the system can identify as being caused by a particular network device. As the network device is the probably the root case, the situation ticket will be routed to the network team.
Instead of investigating each ticket individually, and in silos, the team now has a holistic environmental view of what’s happening in both customer environments and the wider Rackspace environment. The affected teams remain in the loop, but problem solving starts – and more likely ends – with a single team.
The humans on those teams remain the defining factor in any response, but they’re presented with better information so that they can act faster and smarter.
What does AIOps mean for leadership?
Organizations considering augmenting operations with this type of automation capability need to fully understand what that means for the business and its people. It is a decision with implications that run deep into established processes and structures.
On the surface, leaders looking for short-term ROI will see a reduction in labor-per-ticket as a chance to lower headcount. But a longer-term view, and one with a potentially larger payoff, might say that teams now have more time to spend on each issue.
This is the primary opportunity for leadership to enhance people’s position in the post-AI world of work; through upskilling the workforce the presented workload may become more complex. After all, more accurate identification of issues is meaningless without a corresponding improvement in the speed and quality of resolutions.
Marrying AI with automation can reduce the need for human intervention for repetitive issues. A leader may perceive this as an area for full time employee cost reduction but it introduces the need for higher skilled DevOps employees who have deep understanding of technical troubleshooting and can program the automated diagnostic and remediation tasks. Additionally, AI limitations in causal reasoning introduce risk when change actions are unsupervised. The use of supervised machine learning and inclusion of change controls for suggested actions reduces the risks but requires human intervention to provide validation and learning input.
Managers are not immune to change either. When they’re no longer dealing with ticket-crunching queues they will need to transition out of a transactional state and into a project state. And when the system assigns responsibility, managers need to change their working styles, too.
Evolving roles and expectations also necessitate a recalibration of success measures for these teams. The number of tickets closed becomes less relevant when the value is in the nature of that resolution. To reflect this, first-time resolve rate is the defining metric which measures both problem-solving performance and system performance. First-time resolve answers the question: Are we sending issues to the right person the first time? Are they providing valid resolution?
Customer Experience impacts need to be considered. Humans are naturally adverse to change and managing the change through education is essential to the successful introduction of the new operational processes in the organization.
Start small to achieve big
Every company will be different, so a detailed self-assessment is needed to identify initial target areas when considering where and how to start with AIOps. The largest call-drivers are prime candidates, since they promise to have the biggest impact.
For Rackspace, this meant starting with the compute stack and some of the network stack with a roadmap to incorporate the storage stack, public clouds, and the application & security layers. But wherever you start, the key is to establish a foundational position that naturally allows you to build upward through the organization.
In terms of readiness for AIOps, data integrity is key. Machine learning algorithms need to operate on top of trusted data, but there is scope for tolerating some variance. It’s rare for an organization with thousands of devices to have completely accurate data, so you may need to establish a threshold for what’s acceptable. In many cases, if a data set has some variance but is already used on a daily basis, it can continue to be used, but having a plan to further minimize any inaccuracy is suggested.
The people paradox and potential of AIOps
As we’ve seen, the paradox of AIOps is that for an application that promises to help people answer questions, it raises an awful lot of tricky questions for organizations around where and how people fit into their processes and workflows.
What organizations decide to do with the extra human capacity realized by labor-saving machine learning applications anywhere in their organization – not just in IT operations – is going to be critically important to their future success.
Our view is that long-term value creation doesn’t often result from cost-reduction – it’s more likely to come from maximizing the talents that separate humans from machines. AIOps might be the best opportunity in a generation to do just that, by accelerating the creation of a new breed of creative problem-solving IT operations teams.
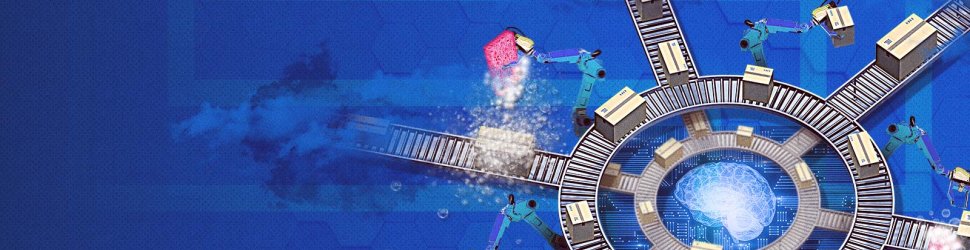
Is Your Customer Data Ready for AI?
About the Authors

Principal Engineer
Jean "JP" Gonzalez
As Principal Engineer at Rackspace JP leads the AIOps vision and strategy for our for Rackspace event and ticketing process. With over 20 years of experience in IT roles across development, management and support services he brings an agnostic perspective to the future of Rackspace infrastructure technologies. JP's passion for efficiency through automation is driving Rackspace approach to operational digital transformation.
Read more about Jean "JP" Gonzalez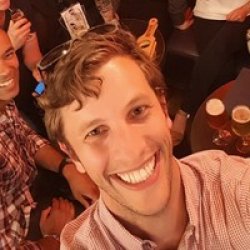
Principal Engineer
Andreas Möller
Andreas is a a talented technical leader with over 20 years of experience working in corporate IT, retail, manufacturing, telecoms, content protection and DRM, disaster recovery and business continuity. A passionate people person that grasps technology, he thrives in pushing unconventional or uncomfortable notions at the start of their life cycles. Frequently called upon for his opinion, he is a natural leader that has recently embraced the notion of algorithmic operations. Andreas is currently a Principal Engineer in Rackspace where he manages a team of custom system engineers along with serving as the chief technical design authority in Rackspace’s EMEA business.
Read more about Andreas Möller