HPC and AI: The IT Power Couple
High Performance Computing (HPC) and AI are revolutionizing industries, from healthcare to finance. With increasing cloud adoption and a focus on greener practices, advancements in HPC and AI will offer improved insights, faster decision-making, and, eventually, greater energy efficiency. This article highlights trends, benefits, and the future of this technological synergy.
AI-driven solutions amplify our capacity to tackle intricate challenges on a vast scale, turning raw data into actionable insights at unprecedented speeds. When businesses combine the capabilities of AI with High Performance Computing (HPC), they are poised to benefit from unparalleled computational and analytical capabilities that pave the way for sharper decisions, heightened efficiency and pinpoint accuracy in planning. HPC and AI are capable of accelerating our problem-solving capabilities while catalyzing groundbreaking innovations in sectors like healthcare, finance and transportation.
Use Cases of HPC and AI
Industries such as healthcare and genomics have long been using HPC for augmented diagnosis and predictive analytics. In finance, HPC assists with real-time stock monitoring and automated trading to determine risk and opportunities. Meanwhile, the oil and gas industry uses HPC to pinpoint reserves, drill for wells and accelerate production.
Many other industries will soon be combining the capabilities of HPC with AI, too. Smart cities will use HPC-powered machine learning to improve traffic management, monitor energy usage, maintain water and air quality and streamline operations. Media, entertainment and design organizations will use HPC for rendering special effects, simulating test scenarios and reducing production costs.
Advantages of using HPC for AI workloads
There are numerous benefits to the integration of HPC and AI solutions. Below are some examples of how this combination can make a real impact today:
- Faster model training: HPC reduces the time it takes to train deep learning models, especially modern architectures with millions or billions of parameters, leading to faster implementation of AI applications.
- Parallelism and concurrency: HPC enables researchers to simultaneously train multiple model configurations, optimizing for recall and precision.
- Improved accuracy: With more computational power, researchers can experiment with more complex models, architectures and techniques, which can lead to improved model performance.
- Improved scalability: HPC offers high-bandwidth, low-latency storage solutions, advanced memory hierarchies, high-speed caches and network interconnects, enabling fast communication for distributed deep learning and parallelized AI workloads.
- Cost savings: HPC has the ability to train models faster and drive innovation, reducing experimentation costs within a short span of time, offsetting the initial investment.
- Streamlined development: HPC environments foster innovation and help cross-pollinate subject-matter experts from different fields, paving the way for easier collaboration.
- Reliability and uptime: Critical applications in healthcare, finance and autonomous vehicles benefit from HPC's redundancy and failover mechanisms, ensuring high uptime and reliability.
The Future of HPC and AI
As technological advancements continue, HPC is becoming more accessible to those organizations that have traditionally had modest budgets, and the demand for HPC in AI-related applications is on the rise. More than ever, HPC and AI will be required to power growth in most industries. Overall, the integration of HPC and AI technologies provides businesses with faster, more precise results, enabling them to make smarter decisions and experience sustainable growth.
HPC in the cloud
Cloud computing facilitates collaboration, scalability and provides security and ease of use for domain experts accessing complex computing architectures, allowing researchers to maximize their investment in computing resources. According to Hyperion, the HPC market is experiencing significant growth and is projected to reach over $50 billion by 2026. Cloud-based HPC is also on the rise, with more buyers shifting from on-premises purchases to the cloud.
The public cloud HPC market is expected to reach $11 billion by 2026, with a significant portion of the revenue being allocated to storage components and the remaining two-thirds spent on compute instances, software licenses, services and other costs associated with running HPC workloads.
Simultaneously, private cloud HPC is becoming increasingly popular for compute-intensive and GPU-memory intensive workloads. It offers benefits such as reduced need for large computing environments, heating, cooling and space. Start-ups and long-established companies are benefitting from the reduced space and cooling requirements derived from HPC architectures.
Most enterprises will find that a combination of public and private cloud solutions will be advantageous for use with static HPC workloads. Running production workloads 24x7x365 on a private cloud can be more cost-effective, while still allowing for burstability and quick experimentation associated with public cloud.
Using a private cloud provides increased security and sovereignty over data, as it allows control over the server architecture and data flow. HPC workloads that cannot be scaled elastically are less expensive to run on a private cloud, making it a viable and cost-effective option for certain use cases.
However, public cloud options for HPC also have their advantages. For example, the Rackspace Data Freedom platform allows users to disaggregate storage from public cloud compute resources, freeing the organization's data from unpredictable and often substantial data-egress fees. This arrangement facilitates the creation of a hybrid solution wherein data can be shared between multiple hyperscale public clouds and a private cloud solution.
Leveraging HPC in the public cloud offers the benefits of a low cost of entry, high availability and easy scalability for variable workloads. Cloud-based services like Amazon EC2 Elastic Compute Cloud, Microsoft’s Azure Batch and Google’s Cloud HPC Toolkit allow users to customize their HPC environments. Open-source offerings like OpenStack are also used to build and manage cloud-based HPC applications.
HPC and your green initiatives
Many would assume that the marriage of green initiatives and HPC has some inherent conflicts, nearly all of which stem from the energy required to drive these solutions. And that assumption is correct. Experts are raising concerns, as in this research study that equates the carbon footprint of a single large natural-language processing (NLP) model to that of five cars over their lifetimes.
But there are numerous approaches to addressing these concerns. On the infrastructure side, maintain your focus on finding efficient cooling solutions. And on the supply side, look into energy providers who can sell you services generated from verifiably renewable sources. You can also heighten your focus on carbon reduction efforts across your organization. These efforts might include measuring and tracking your carbon footprint, reducing consumption in your data center or reevaluating your storage footprint.
HPC milestones we’ll see in the near future
Looking ahead, we expect to see several new developments in HPC, including:
- Exascale computing: The Fronter HPE Cray supercomputer at Oak Ridge National Laboratory became the first to hit the exaflop scale in 2021. Exaflop refers to computing systems capable of executing one quintillion floating-point operations per second.
- Advanced architectures: Continued innovation in integrating CPUs, GPUs and other accelerators like TPUs and FPGAs. Chiplet-based designs allow modular components to be combined on a single package, simplifying distributed computing requirements for large AI models.
- AI optimized hardware: More HPC solutions are featuring AI accelerators in chips and networks for deep neural network training and inferencing.
- Energy efficiency: As mentioned above, with the increase in power of HPC systems, there is a need for greater energy efficiency. Measures such as using low-power GPUs, advanced cooling systems, renewable energy sources, compressing the number of cores on a chip, and developing software and AI models that require less compute will contribute to greener HPC solutions.
- Software innovations: Improved hardware and energy efficiency drive the development of easy-to-use software and distributed computing paradigms, enabling the creation of new solutions that were previously not possible.
- Quantum computing: Breakthroughs in developing fault-tolerant qubits are expected to lead to usable quantum systems that can integrate with or replace traditional HPC environments.
Overall, the future of HPC is set to witness advancements in performance, energy efficiency, AI integration and software innovation, ultimately enabling more powerful and sustainable computing solutions.
Capitalize on the power of AI, quickly and responsibly with Foundry for AI by Rackspace Technology (FAIR™).
FAIR™ is at the forefront of global AI innovation, paving the way for businesses to accelerate the responsible adoption of AI solutions. FAIR aligns with hundreds of AI use cases across a wide range of industries while allowing for customization through the creation of a tailor-made AI strategy that’s applicable to your specific business needs. Capable of deployment on any private, hybrid or hyperscale public cloud platform, FAIR solutions empower businesses worldwide by going beyond digital transformation to unlock creativity, unleash productivity and open the door to new areas of growth for our customers. Learn more →

Take the first step toward understanding the growing impact of HPC and AI here.
About the Authors

Chief Architect - Data & AI
Nirmal Ranganathan
Nirmal Ranganathan is the Chief Architect – Data & AI at Rackspace Technology and responsible for technology strategy and roadmap for Rackspace's Public Cloud Data & AI solutions portfolio, working closely with customers, alliances and partners. Nirmal has worked with data over the past 2 decades, solving distributed systems challenges dealing with large volumes of data, being a customer advocate and helping customers solve their data challenges. Nirmal consults with customers around large-scale databases, data processing, data analytics and data warehousing in the cloud, providing solutions for innovative use cases across industries leveraging AI and Machine Learning.
Read more about Nirmal Ranganathan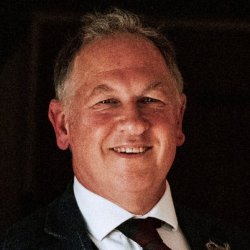
CTO for EMEA
Simon Bennett
As Chief Technology Officer for EMEA regions at Rackspace Technology, Simon’s goal is to deliver end-value to customers with world-class multicloud solutions and services. He is focused on inspiring and supporting technical leaders to find the best-fit solutions combining a perfect blend of services and capabilities from the Rackspace Technology and partner portfolio. Simon’s extensive experience has been gained from working across a broad spectrum of industries. Simon previously worked for IBM for just over 20 years in leadership roles. Most recently, he provided technical pre-sales and detailed solution support for strategic deals within technology services.
Read more about Simon BennettRelated Topics