Highlights from Swami Sivasubramanian's AWS re:Invent 2023 Keynote
by Masoom Tulsiani, AWS Cloud Architect, EMEA Professional Services, Rackspace Technology

The symbolic relationship between humans and AI is similar to the symbolic relationship between remoras and manta rays, according to Swami Sivasubramanian, Vice President of Database, Analytics and Machine Learning at AWS. He gave a keynote address at AWS re:Invent 2023 in Las Vegas in November.
For those not familiar, remoras cling onto manta rays for protection, transportation and scraps from manta rays’ meals. Remoras benefit from manta rays by cleaning their skin of bacteria and parasites.
Sivasubramanian’s session began with Amazon Web Services (AWS) showcasing its AWS Machine Learning Infrastructure, including its data compute stack and layers. AWS now supports the latest models from Ai21 Jurassic-2, Anthropic, Cohere, Meta and Stability AI, as well as additions to the Amazon Titan models — the models of choice for many organizations.
Attendees also learned that:
- Running cost-effective infrastructure remains a challenge
- Data is still a differentiator for organizations
- The success of generative AI applications will depend on how effectively companies can train their language models
Six key highlights
Among the many announcements throughout the event, six key highlights stole the spotlight:
- Amazon Titan image generation in Amazon Bedrock: Now, AWS users can generate realistic, studio-quality images at large volume and low cost using natural language prompts in Amazon Bedrock. Also, AWS’s Generative AI Innovation Center will be providing AI certifications and helping customers with their Bedrock generative AI applications.
- Anthropic's Claude 2.1 and Meta's Llama 2 70B models: Both are available now on Amazon Bedrock and suitable for large-scale tasks, such as language modeling, text generation and dialogue systems. Claude 2.1 offers a 200K token context window and improved accuracy in long documents.
- Amazon Titan Multimodal Embeddings: Allows organizations to build more accurate and contextually relevant multimodal search and smart recommendation experiences. The model converts images and short text into embeddings — numerical representations that allow the model to easily understand semantic meanings and relationships among data. These are stored in a customer’s vector database. Read more about this innovation here.
- Amazon SageMaker HyperPod: Helps reduce time-to-train foundation models (FMs) by providing a purpose-built infrastructure for distributed training at scale, helping to reduce the time it takes to train models by up to 40%.
- Model Evaluation on Amazon Bedrock: Facilitates access to curated datasets and predefined metrics for automatic evaluations, helping to evaluate, compare and select the best foundation models for AI use cases.
- AWS Clean Rooms ML: Helps users apply machine learning models to generate predictive insights without sharing underlying raw data. Also specifies training datasets using the AWS Glue Data Catalog.
Sivasubramanian demoed the model editing feature using the Amazon Bedrock image playground with the Amazon Titan image generator. It generated background variations via prompts and incredible image-generation features.
Database and analytics services
Attendees were also excited to hear about the following data and analytics announcements from Sivasubramanian:
- Amazon Neptune analytics: An analytics database engine that can help surface insights by analyzing tens of billions of connections in seconds with built-in graph algorithms, enabling faster vector searches with both graphs and data.
- Amazon Q generative SQL for Amazon Redshift Serverless: Enables data engineering teams to accelerate data pipeline build. Q can write SQL queries faster using natural language and help simplify the process for custom ETL jobs.
- Amazon OpenSearch Serverless vector engine: This will lead to more efficient searches and processes.
- Amazon DocumentDB and Amazon DynamoDB Database vector capabilities: Coming soon to allow users to store multiple kinds of data together.
- Amazon MemoryDB for Redis: This will support vector search, leading to faster response times and allowing tens of thousands of queries per second. It’s a useful application, in particular, for fraud detection in financial services.
- Databases like MongoDB and key-value stores like Redis: These will be available as a knowledge base in Amazon Bedrock.
- Amazon Q data integration: This will be available in AWS Glue.
AWS partners share AI insight
Attendees also got real-life use cases from partners like Booking.com and Intuit. Rob Francis, CTO of Booking.com, discussed how the company built its AI Trip Planner application by hosting Llama 2.0 and using Amazon Sagemaker. The key components of its recommendation API use Amazon Bedrock and Titan.
Nhung Ho, VP of AI at Intuit, shared insights on his experience building a tool called GenX to deploy generative AI experiences with the help of a scalable architecture built on SageMaker, Bedrock and Redshift. His team also built Intuit Assist, which uses financial large-language models (LLMs) to deliver insight on topics like personal finance.
To wrap things up, it’s important to mention that to get the most out of generative AI, Sivasubramanian emphasized how critical it is to have a strong data foundation that includes data security. This is particularly important because we’re all experiencing an explosion of data. AWS is thinking about this with every innovation. For example, Titan-generated images have watermarks to help reduce the spread of misinformation.
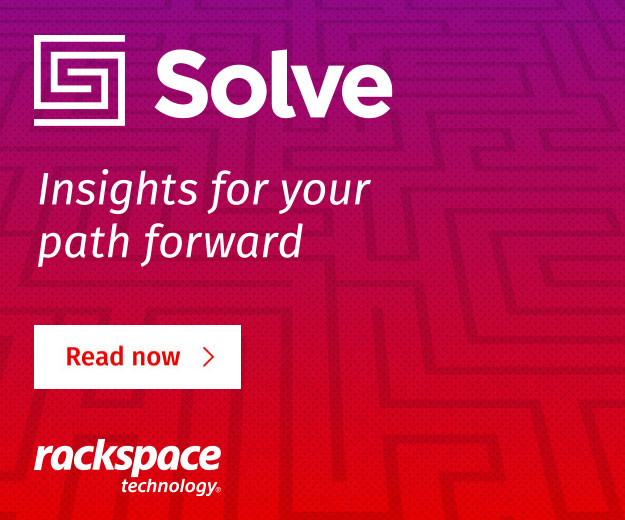
Recent Posts
Highlights from Dell Technologies World 2024
June 5th, 2024
Driving Innovation into Today’s Automotive Market: Powering Stronger Customer Experiences
May 2nd, 2024
Google Cloud Next ’24 Highlights
April 23rd, 2024