AI and machine learning are revolutionizing modern businesses — here’s how to get ahead
Pierre Fricke

Fierce competition means every business must adapt to succeed. AI and machine learning have emerged as modern, vital ways for organizations to get ahead. Many businesses today prioritize data, analytics and AI/machine learning projects to power new business models, enhance product and service offerings, improve efficiency, drive revenue and deliver superior customer experiences.
But analyst figures on project implementation make for sobering reading. Gartner predicts that under half of modern data analytics and machine learning projects will be successfully deployed in production by 2022. Less than a fifth will move piloted AI projects into production without delays caused by a range of problems — from technical skills gaps and lack of IT/business process maturity, to insufficient organizational collaboration.
For example, these businesses may not have expertise in mathematics, algorithm design or data science and engineering. Or their data may not be in a unified data lake infrastructure for ready access. These conditions create challenges for any organization looking to advance in the market and derive value from AI and machine learning.
This combination of pressure and challenges can overwhelm your business, especially if you’re at the start of your AI and machine learning journey. So let’s dig into why your business should make the effort — and how doing so might require different skills sets and data from what you might think.
What are AI, machine learning and deep learning?
Let’s start with the basics. When a machine completes tasks based on a set of stipulated rules that solve problems, we’re into the realm of artificial intelligence. This might include understanding and interpreting natural language, recognizing when objects move and providing intelligent answers. Business benefits follow, such as analyzing data sets that are too large for humans to process, answering questions in real time that draw from existing data and experiences, and automation that can reduce costs and boost productivity.
Machine learning is a discipline within the AI domain. It enables machines to learn by themselves using data. They use this knowledge to make increasingly accurate predictions and drive actions. For this to happen, you need a model that’s trained on existing data, after which point it can process additional data and make predictions. Throughout the process, it’s important to track and understand your model, building quality and eliminating bias.
Finally, deep learning is a subfield of machine learning. It structures algorithms in layers to create an artificial neural network that can learn and make intelligent decisions on its own.
The many use cases of AI and machine learning
We’ve so far explored AI, machine learning and deep learning in the abstract, but in what specific ways can they benefit your business?
- Answering questions, thereby improving customer support and buying journeys, through faster, higher-quality answers and experiences.
- Speech recognition, including text-to-speech and speech-to-text translation, enabling you to work with voice/audio data more widely and productively.
- Document summaries that effectively extract key concepts to use in countless ways, improving productivity and document data use.
- Image recognition for biotech, satellite/drone imagery and face recognition, to quicken emergency responses and prevent crime.
- Image processing to improve the presentation and utilization of images through enhanced resolution and colorization.
- Data classification in medicine, to yield better diagnoses, faster and more targeted treatments and health preservation.
- Superior search to get customers to what they want more rapidly, whether that’s a product recommendation or a web page.
- Strategic analysis that can be a boon to the games industry, driving more challenging and educational entertainment.
- Financial and logistical forecasting to improve financial management, planning and resource allocation/utilization.
AI and machine learning require skills and data, but not what you think
If you’re looking to machine learning and deep learning but have concerns about your existing data, be mindful that they don’t always need massive data sets. While completely new models with no data nor training do require tens of thousands to millions of data points, trained models exist that can give a project leader a head start. Even if you have just 100 or so examples for a specific use case, building on a general model’s foundation could yield more accurate results than human experts would provide.
Additionally, it’s worth thinking differently about hiring for the delivery of AI/machine learning enabled applications and solutions. There’s an assumption you need PhD-level data scientists. Although they do add value and can be necessary in some circumstances, existing staff can often be trained in about 100 hours, building on high-school math and a year of coding experience. With modern tools on AWS or Google Cloud including AutoML, they can build the solutions you need.
In all, it’s as much about changing your mindset as anything else. You must think about what AI and machine learning can bring to your business and the most effective way to achieve that, thereby keeping your company ahead. Machine learning is today driving change in thinking of data as code — where machine learning uses data to write the program, which is the output.
This methodology coupled with the tools and education I mentioned earlier set the stage for many more people collaborating to fashion a new generation of intelligent solutions that will revolutionize business for years to come.
For more information on AI and machine learning, check out our panel discussion, which dives deep into these topics. The discussion covers: toolsets and methodologies; capabilities and constraints; data, computer and expertise requirements; examples of successful applications; and how to get started.
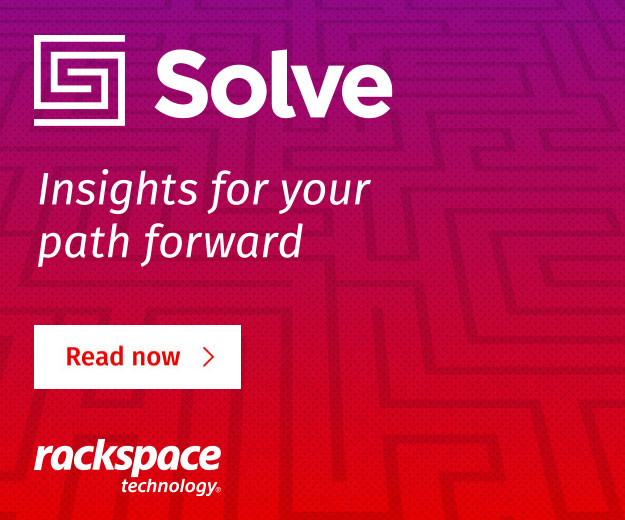
Recent Posts
Highlights from Dell Technologies World 2024
June 5th, 2024
Driving Innovation into Today’s Automotive Market: Powering Stronger Customer Experiences
May 2nd, 2024
Google Cloud Next ’24 Highlights
April 23rd, 2024