Is Your Customer Data Ready for AI?
Sales reps only spend a third of their time actually selling. Can AI help to maximize their time?
According to research from Salesforce, sales reps only spend a third of their time actually selling. Instead, 40% of their time is spent on activities like manually entering sales info, generating quotes or proposals and completing administrative tasks. These activities distract from relationship building and introduce inefficiencies into the quote-to-cash (QTC) cycle. The QTC cycle enables the entire sales process — from stimulating a buyer’s interest through realizing revenue from a purchase. However, the path is often hindered with siloed technology, disjointed teams (sales, marketing, accounting, support) and outdated processes.
Enter artificial intelligence (AI) and other predictive frameworks. Layering these technologies on top of the sales cycle can shorten the path to closing sales, improve the quality of sales pipelines, offer deeper insights into prospects and give sales and support more time to build relationships. According to Gartner, AI produces up to 30% higher conversion rates when engaging with leads. And the Harvard Business Review predicts that AI can create over $2 trillion of value in the marketing and sales chain. In the time it takes a sales person to do hours of research to vet a prospect, AI-assisted automation tools can evaluate and organize thousands of prospects to allow the rep to focus on selling instead of research.
With so much to gain, what could go wrong?
If data that informs AI is siloed, biased, inaccurate or incomplete, AI is useless. It can’t deliver the right insights and may even do the opposite by surfacing faulty conclusions that hinder, instead of carrying the sales process forward.
The success of AI and other predictive frameworks relies on the veracity of the data. Garbage in means garbage out. Without solid integration between applications and accurate data, attempts to leverage any of these frameworks in the sales cycle will result in failure. For the algorithms to analyze a specific trend or make an intelligent recommendation, it needs access to as much good data as possible. Up to 80% of the work involved in an AI project is focused on data collection, cleaning and preparation.
However, properly integrated, clean data powered by AI capabilities can make a dramatic impact on sales revenue. Airline KLM has generated millions in added revenue by using AI frameworks to give agents more time to help customers book upgrades and new flights. Caesars, the hotel and casino group, uses AI to analyze customers’ potential daily spending to customize and prioritize interactions and promotions. And for their hotel guests, predictive customer service tools have reduced calls to the front desk by 30%. Using an AI-powered recommendation engine, Netflix claims to save $1 billion annually by avoiding cancelled subscriptions. The foundation of these AI successes isn’t just the application of the AI technology, but also the quality of the underlying customer data and the private, public or hybrid cloud environments where the data lives.
As you begin to strive toward your AI ambitions, establish clear success factors. Carefully balance industry benchmarks, risk and business needs to guide what counts as a win. Keep in mind that industry benchmarks are just that – benchmarks. Use them to guide your steps, but not to order your steps. They can only confirm that you’re doing what everyone else is doing, but not how that aligns with your business goals. Based on the risk involved in your AI endeavors, following benchmarks too closely could be detrimental. For example, if your AI application is related to life-threatening processes like health care or self-driving cars, your benchmarks may be out of line with the industry, but in line with the added care and risk needed to avoid harm.
Don’t let dirty data derail your AI journey
AI will happily train on bad data, and it will happily give you bad results. Before you start planning your AI initiatives, focus on ensuring that your existing data is ready to take advantage of deep processing capabilities. 52% of CRM data is erroneous. From legacy systems that hold critical data, to remnants from mergers and acquisitions, to dirty data taking up space, corralling and organizing data within the individual systems can be a challenge.
So, should you clean up the data you’ve already collected or start from scratch? AI thrives on vast buckets of data. To get meaningful insights, you’ll need at least 10,000 data points. Experts agree on the rule of 10: For each data point measured, you need 10x data to produce a useful inference. Starting over and amassing tens of thousands of clean data points is likely impractical, and it means ignoring the rich set of data that you already have. At first glance, it may feel like an overwhelming task, but the work you put into cleaning your data now will result in more precise insights later.
One of the biggest dirty-data culprits is duplicate records. There are several third-party and in-app tools to help you merge records and identify duplicates. To further streamline the data that your sales team is using, integrate a process for archiving inactive records. And if you haven’t already, standardize your data-entry practices. The more you change the variables, the less valuable the data are for analysis. Badly formatted data may not feed into search and other data-gathering functions. Give users prompts or guides for formatting customer names, addresses, product information and other vital data. And once you’ve done a complete audit and cleanup of your application data, set a regular maintenance schedule to address ongoing data hygiene.
Finding the right approach to integration
In the 2019 Data Science and Machine Learning Market Study Report, 40% of marketing and sales teams identify AI and machine learning as critical to their success. One of the best ways to see the full picture of your customer data is to integrate your front end (CRM) and back end (ERP) systems. By integrating your existing ERP and CRM system data, you can create a data repository that forms the groundwork for future AI initiatives. Here are four common ways to achieve tighter integration between ERP and CRM, ranging from the most complex to the most efficient:
- Swivel chair: This manual process requires a member of your team to manually transfer data from one system to another.
- First-party tools: This method relies on various features and options included with one or more of the apps in your QTC path.
- Point-to-point integration: This integration method leverages APIs to directly connect your systems to share and utilize data.
- Middleware ETL: Servers that you host and maintain to are used to facilitate sharing between software and applications.
Each of these options comes with tradeoffs in complexity, efficiency and cost. Addressing data hygiene before applying AI frameworks increases your chances of leveraging the full power of predictive technologies so that sales and support can stay focused on building relationships and generating revenue.
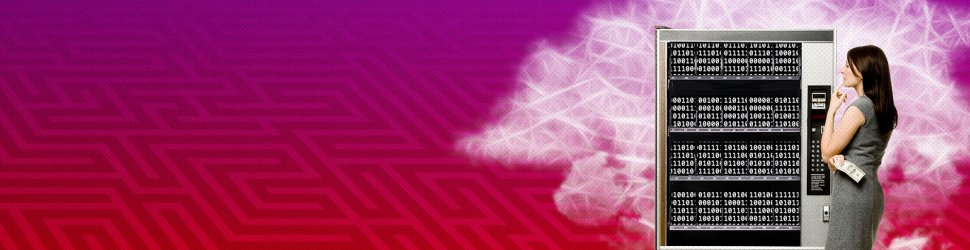
How Intelligent Data Addresses the Chasm in Cloud
About the Authors
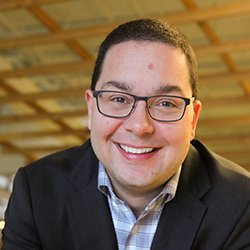
CTO
Tolga Tarhan
As CTO of Rackspace Technology, Tolga Tarhan leads the vision, driving innovation, and strategy for our technology offerings. With more than two decades of experience leading product and engineering teams and as a hands-on technologist at heart, he brings unique insights to customers undertaking the journey to the cloud. As an early pioneer of cloud native thinking, Tolga's passion has driven our technical approach and transformed our customers into cloud native thinkers. Tolga continues to show thought leadership in the field through his extensive speaking engagements at AWS events, industry conferences, and educational groups. Tolga previously served as CTO of Onica, which was recently acquired by Rackspace Technology. Prior to that, he was a co-founder of Sturdy Networks and served as the CEO through to the acquisition by Onica. Tolga holds an M.B.A. from the Graziadio Business School at Pepperdine University.
Read more about Tolga TarhanRelated Topics