Struggling to Fully Power Data Analytics? Decentralization Might Be the Problem.
Decentralizing data analytics is all the rage. But is it all it’s cracked up to be?
It’s commonly accepted that if you want to build a data-driven culture, you need to decentralize data analysis and decision-making. After all, business units are closer to the end customer. Giving them the tools they need to analyze data and generate insights on their own should enable more agility and greater responsiveness to customer needs.
Or at least that’s the theory. In my experience, decentralizing data analysis does more harm than good. By fragmenting your infrastructure, you introduce redundancies and disconnects that keep you from harnessing the full power of your data at any level.
Instead of decentralizing by default, organizations should consider whether the thoughtful centralization of data analysis will drive stronger results for their businesses.
Left in the dark by decentralization
Despite the trendiness of “decentralized data,” few organizations ever totally decentralize their data governance structures. It’s usually just the analysis and decision-making functions that get distributed across business units, while some central authority such as a Center of Excellence (CoE) remains responsible for ensuring data integrity and quality across the organization.
However, centralizing governance alone isn’t enough to prevent fragmentation. When each business unit is empowered to build out its own data analysis teams, processes and infrastructure, it impedes the CoE’s visibility into how data is used across departments. Eventually, this creates major disconnects.
Similarly, organizations that decentralize data analysis can easily end up data rich, but insight poor. Disconnected from a central strategy, data initiatives by individual business units lack power and fail to generate results. This fragmentation can also make it difficult to get a clear picture of basic business metrics, which may be calculated in different ways by different departments. For example, before I led the centralization of Rackspace Technology data analytics, we were tracking more than 10 different versions of net promoter score (NPS) and churn across all our departments.
These inefficiencies are bad enough when you’re trying to execute on everyday tasks. But if they want to build a data-driven culture, organizations need to be developing next-generation capabilities like cloud-based analytics, as well as driving data literacy and facilitating change management. These important components of a successful data strategy are often overlooked when organizations plan for the future — and they’re much easier to achieve when you harmonize your data through a centralized function.
Turning the lights on with centralization
When you centralize data analysis, it’s like ensuring that every room gets power from the main electrical grid. The lights go on across the house. More importantly, you can remodel the building however you want, because you know how the wiring is connected from room to room. Change management becomes much easier, because you have that governing body that can become the catalyst for driving a data-driven culture.
At Rackspace Technology, transitioning from a decentralized model to a centralized one has actually increased our agility. We’ve increased efficiency to the point that we get six times the work out of the same size team. Centralization doesn’t mean sacrificing data democratization, either. Since I joined the company a year and a half ago, the number of employees using data in their day-to-day work has increased more than 10x.
Digital transformation isn’t one-size-fits-all, and there may be some cases where decentralization makes more sense for a company. But in my experience, most organizations benefit from centralizing data analytics and decision-making under a central data office. If you’re ready to make the transition, there are a few considerations you should keep in mind:
- Start with business goals. The ultimate goal of any data initiative is to serve the business, so focus your centralization efforts by identifying the top strategic priorities at your company. At Rackspace Technology, our top goals were to improve customer experience and reduce churn, which was why my first data initiative focused on NPS.
- Organize a knowledge exchange. The next step is to start building a holistic view of how data is used across the organization. Which metrics are being tracked, and how are they being calculated? What tools does each department use? Start with the use cases that are most closely tied to the business goals you identified in the first step.
- Centralize staffing. Plan to reabsorb the data analysts currently scattered across departments into your central data office. Design their new roles to leverage the domain expertise they’ve gained while working closely with business units. At Rackspace Technology, we trained many of these team members on data visualizations and storytelling, and put them to work as consultants who spend their time brainstorming solutions to business problems.
Lighting the way to future insight
No one would build a house with “decentralized” electrical wiring — and if they did, it wouldn’t be up to code. So, why are so many organizations choosing to decentralize their data analytics? If you’re struggling to get the results you need out of your data programs, consider bringing analytics and decision-making across your organization under a centralized data office. It may be all you need to finally turn all the lights on — and benefit from increased efficiency and faster, more useful insights for all your departments.
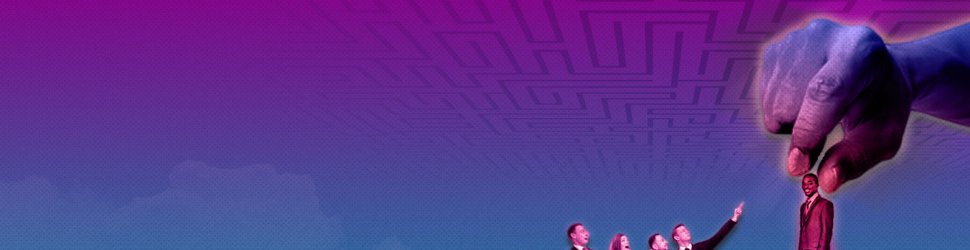
Traditional vs. Modern CDO — Breaking Down the Changing State of the Chief Data Officer
About the Authors

Chief Information Officer
Juan Riojas
As Chief Information Officer at Rackspace Technology, Juan Riojas is responsible for enterprise-wide data strategy, management, and analytics to meet the need of the business to answer critical questions through time to insight. He has more than 20 years of industry experience successfully migrating data ecosystem across all public clouds, leading to significant business transformation outcomes. Prior to Rackspace, Juan worked for Informatica building their inaugural Data Office and has held various executive leadership roles at Gogo, Dell, Accenture, and Expeditors. A native of Texas, Juan attended Texas A&M International University, where he studied business administration and holds a post graduate degree from Said Business School, Oxford University
Read more about Juan Riojas