Gotta Keep ‘em Separated: Why AIOps and MLOps Aren’t the Same Thing
AIOps and MLOps have similar names but they solve very different challenges.
Tech leaders hunting for greater operational efficiencies are increasingly turning to automation, leading many to explore what AIOps and MLOps can do for them. And something they’ll discover quickly is that, while they have similar names, AIOps and MLOps very different disciplines and technologies.
AIOps is about increased efficiency in IT operations, achieved by automating incident/management diagnostics and intelligently finding the root cause through machine learning. By sifting through the noise generated by monitoring systems and reducing the false positives, these solutions present technical teams with high quality information that is easy to understand, so that they can get to work on a resolution.
MLOps, on the other hand, focuses on creating an automated pipeline for bringing machine learning models into production. It looks to overcome the disconnect between data science or data ops teams and infrastructure teams, to get models into production faster and more often. Importantly – and in contrast to AIOps – MLOps doesn’t directly refer to a machine learning capability per se, with algorithms processing data. Rather, it’s a way to manage and streamline the building, deployment and maintenance of those algorithms.
However, despite the stark differences between the two, there are overlaps in the skills, teams and mindsets required to successfully adopt AIOps and MLOps. This will prove an advantage for tech leaders if – as we expect it will – interest in each technology continues to grow. So it’s worth diving deeper into where they overlap, as well as offering some do’s and don’ts when it comes to adopting AIOps and MLOps based on our experience working with customers.
Many enterprises already have the foundation for both AIOps and MLOps
AI, of which machine learning is just one application, is not generally a mature discipline within businesses. But many of the skills needed to begin experimenting with it for either AIOps or MLOps have been around for a while.
Let’s start with AIOps. Building models that can automate systems monitoring and output intelligent failure reports or alerts first requires experienced DevOps personnel — engineers and data analysts. It also requires operations administrators with deep subject matter expertise around the processes you’re analyzing for automation and the adjacent workflows that they influence or impact.
To then deploy those models into production, operational AI expertise is required. These specialists are much harder to come by, of course. But their contribution is vital when it comes to helping the engineering teams build out event correlations within their models. These specialists are also invaluable when it comes to feeding those models with data to train them and then keep them updated as their operating environment changes. (Contrary to popular belief, AI doesn’t build or maintain itself; it needs a lot of human intervention and direction to understand which correlations are important and respond to changes.)
Building an MLOps deployment pipeline for your models requires all of the above, plus personnel that have infrastructure knowledge overlapped with both some data science understanding and some machine learning engineering experience. Experts in all three likely don’t exist, but people or teams with understanding of each are essential.
Larger organizations have an advantage in that, generally, they tend to have these skills already. It’s mostly a matter of finding a way to combine them. A further advantage is the fact that they also have the budget and resources to seek the external help they will almost certainly need, particularly on the AI side, in the shape of consultants or even academics.
As usage of AI matures, we expect to see these larger organizations begin to connect a lot of these components and people together, and retitling them. For example, it’s easy to imagine dedicated MLOps teams emerging, featuring a mix of data science and infrastructure personnel, as businesses expand their capabilities and investments in machine learning. Among the responsibilities for these teams would be getting AIOps into production.
The do’s and don’ts for building these foundations into an AIOps or MLOps capability
An AIOps or MLOps project will never be an easy lift, even with these foundations of skills and personnel in place. In our experience, high-level do’s and don’ts would include:
- Don’t start too large: Starting with a smaller target that closely fits your understanding, capabilities and resources will allow you the space to test and refine both the technology and new team structures, before broadening your ambitions.
- Don’t reinvent the wheel: There’s already a big market for AIOps and MLOps solutions and a thriving open source community. Prebuilt models for your use case likely exist already, and these can be trained for your needs and based on your data through a process called transfer learning. We recommend leveraging this wealth of existing research and solutions.
- Don’t create unattainable expectations: MLOps and AIOps will not solve your problems in a day, or even a quarter. It’s important to create and manage appropriate expectations at the leadership level, around both time to impact and return on investment. Adopting any AI application is a long-term play. There’s a high ceiling on possible gains, but patience is essential given the process and organizational changes that are required — not to mention the steep technological learning curve.
- Do assign clear responsibilities: This is vitally important once you start mixing and matching people into new teams with new deliverables.
- Do monitor for model and data drift: The common assumption that AI can somehow look after itself is not only wrong, it’s also operationally and reputationally risky. Performance of all models degrades over time as the environments they’re monitoring change for whatever reason (new products and personnel are introduced, or simply through the unintended consequences of seemingly unrelated process changes). Your AIOps and MLOps protocols must account for this.
- Do measure performance and react to change: It’s vital to know what success looks like for your AIOps and MLOps models and processes, and to attach metrics to those outcomes that can be monitored and responded to.
- Do have in place strong governance and auditing processes: This is a huge challenge relating to AI in general right now. When machines make decisions that impact the business, its people or its customers, those decisions must be explainable – and contestable if necessary. Governance and auditing begin with a focus on transparency as we build models, and runs through to strong oversight of the decisions made and their outcomes once in production.
- Do respect data integrity: It’s well known that quality data is the backbone of success with AI. It defines the design of your models and systems and the success of their outputs: if your data is off, then everything is off. An often overlooked quality factor, however, is human bias. Many systems have human-driven data inputs, and that data will bring with it conscious or unconscious biases reflecting assumptions about its correlations. To guard against this, your processes must have a trigger or workflow step that prompts a correction of the data when needed.
What are the future growth prospects for AIOps and MLOps in the enterprise?
While as an industry we know much more about what it takes to succeed with AI and machine learning than we did just a couple of years ago, an honest view of the landscape has to conclude that it is only really being dabbled in right now.
Things have certainly moved beyond the realm of “buzz,” and a handful of businesses have shown that AI has real enterprise applications that can generate tremendous value. As more businesses, inspired by this success, become involved, they will recognize pretty quickly that they need an MLOps solution if they’re to reliably see a return on their investment. As interest in machine learning grows, interest in MLOps can only grow with it.
On the other hand, AIOps is more advanced in its penetration of enterprises – but it is, and will likely remain, the preserve of large organizations with their own IT teams. These companies have the most to gain from improved process efficiencies and the greatest scope to redeploy operational resources to more value-add activity. And we’re yet to meet an enterprise that doesn’t covet either of these outcomes.
So, while not getting the two technologies confused is something of a 101 lesson, the overlap in skills means that tech leaders can reasonably expect to be able to accommodate both on their transformation roadmap. And we expect that most will, sooner rather than later.
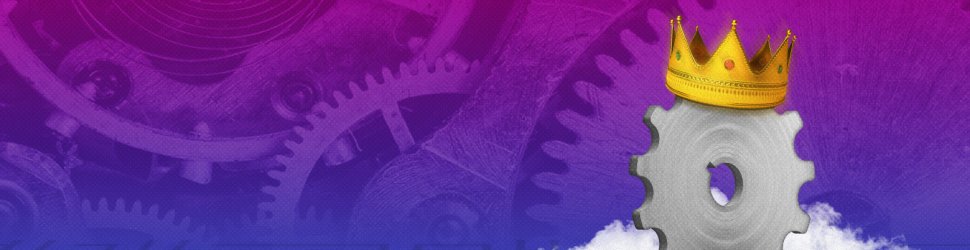
Cut Straight to the Tech Trends that Matter
About the Authors
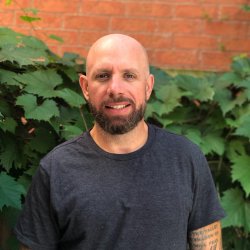
Practice Manager, Data Science & Engineering
Mark McQuade
Mark McQuade is an AWS and cloud-based solution specialist, knowledge addict and relationship builder. Earlier in his career, Mark held technical support, operations, business development and leadership roles for a telecommunications solutions provider, where he worked for 13 years. He then transitioned to the world of cloud and opened up his own AWS small business before joining Onica, who was acquired by Rackspace technology in 2019. Mark is currently Practice Manager of Data Science & Engineering at Rackspace Technology. Every day, he gets to learn more about what he is passionate about professionally – AI and machine learning – as well as the fascinating world of data. As a technology evangelist, you’ll often find Mark promoting data and AI/ML at talks, webinars, podcasts and industry events.
Read more about Mark McQuade
Principal Engineer
Jean "JP" Gonzalez
As Principal Engineer at Rackspace JP leads the AIOps vision and strategy for our for Rackspace event and ticketing process. With over 20 years of experience in IT roles across development, management and support services he brings an agnostic perspective to the future of Rackspace infrastructure technologies. JP's passion for efficiency through automation is driving Rackspace approach to operational digital transformation.
Read more about Jean "JP" GonzalezRelated Topics